by Sara Rebein, Leibniz-Institut für Analytische Wissenschaften – ISAS – e. V.
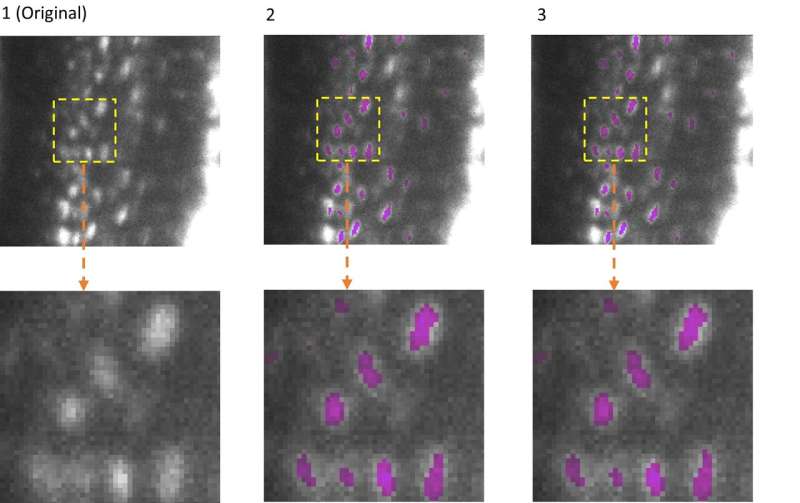
Artificial intelligence (AI) has become an indispensable component in the analysis of microscopic data. However, while AI models are becoming better and more complex, the computing power and associated energy consumption are also increasing.
Researchers at the Leibniz-Institut für Analytische Wissenschaften (ISAS) and Peking University have therefore created a free compression software that allows scientists to run existing bioimaging AI models faster and with significantly lower energy consumption.
The researchers have presented their user-friendly toolbox, called EfficientBioAI, in an article published in Nature Methods.
Modern microscopy techniques produce a large number of high-resolution images, and individual data sets can comprise thousands of them. Scientists often use AI-supported software to reliably analyze these data sets. However, as AI models become more complex, the latency (processing time) for images can significantly increase.
“High network latency, for example with particularly large images, leads to higher computing power and ultimately to increased energy consumption,” says Dr. Jianxu Chen, head of the AMBIOM—Analysis of Microscopic BIOMedical Images junior research group at ISAS.
A well-known technique finds new applications
To avoid high latency in image analysis, especially on devices with restricted computing power, researchers use sophisticated algorithms to compress the AI models. This means they reduce the amount of computations in the models while retaining comparable prediction accuracy.
“Model compression is a technique that is widely used in the field of digital image processing, known as computer vision, and AI to make models lighter and greener,” explains Chen.
Researchers combine various strategies to reduce memory consumption, speed up model inference, the “thought process” of the model—and thus save energy. Pruning, for example, is used to remove excess nodes from the neural network.
“These techniques are often still unknown in the bioimaging community. Therefore, we wanted to develop a ready-to-use and simple solution to apply them to common AI tools in bioimaging,” says Yu Zhou, the paper’s first author and Ph.D. student at AMBIOM.
Energy savings of up to roughly 81%
To put their new toolbox to the test, the researchers led by Chen tested their software on several real-life applications. With different hardware and various bioimaging analysis tasks, the compression techniques were able to significantly reduce latency and cut energy consumption by between 12.5% and 80.6%.
“Our tests show that EfficientBioAI can significantly increase the efficiency of neural networks in bioimaging without limiting the accuracy of the models,” summarizes Chen.
He illustrates the energy savings using the commonly used CellPose model as an example: If a thousand users were to use the toolbox to compress the model and apply it to the Jump Target ORF dataset (around one million microscope images of cells) they could save energy equivalent to the emissions of a car journey of around 7,300 miles (approx. 11,750 kilometers).
No special knowledge required
The authors are keen to make EfficientBioAI accessible to as many scientists in biomedical research as possible. Researchers can install the software and seamlessly integrate it into existing PyTorch libraries (open-source program library for the Python programming language).
For some widely used models, such as Cellpose, researchers can therefore use the software without having to make any changes to the code themselves. To support specific change requests, the group also provides several demos and tutorials. With just a few changed lines of code, the toolbox can then also be applied to customized AI models.
About EfficientBioAI
EfficientBioAI is a ready-to-use and open-source compression software for AI models in the field of bioimaging. The plug-and-play toolbox is kept simple for standard use, but offers customizable functions. These include adjustable compression levels and effortless switching between the central processing unit (CPU) and graphics processing unit (GPU).
The researchers are constantly developing the toolbox and are already working on making it available for MacOS in addition to Linux (Ubuntu 20.04, Debian 10) and Windows 10. At present, the focus of the toolbox is on improving the inference efficiency of pre-trained models rather than increasing efficiency during the training phase.
More information:
Yu Zhou et al, EfficientBioAI: making bioimaging AI models efficient in energy and latency. Nature Methods (2024). www.nature.com/articles/s41592-024-02167-z
EfficientBioAI is available at github.com/MMV-Lab/EfficientBioAI
Provided by
Leibniz-Institut für Analytische Wissenschaften – ISAS – e. V.
Citation:
Analyzing microscopic images: New open-source software makes AI models lighter, greener (2024, January 24)
retrieved 30 January 2024
from https://techxplore.com/news/2024-01-microscopic-images-source-software-ai.html
This document is subject to copyright. Apart from any fair dealing for the purpose of private study or research, no
part may be reproduced without the written permission. The content is provided for information purposes only.
______
Source : https://techxplore.com/news/2024-01-microscopic-images-source-software-ai.html